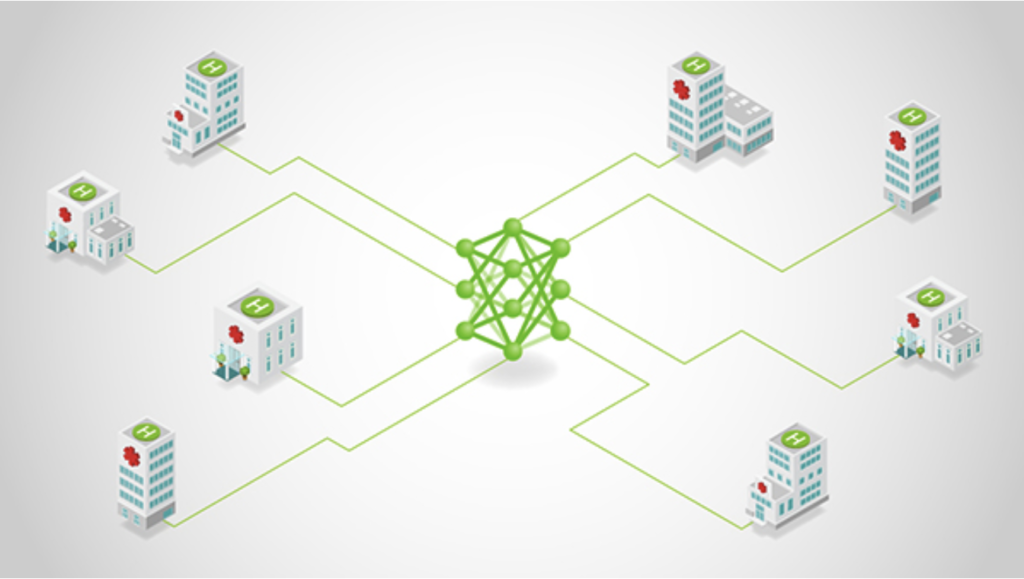
NeuFL®
Train your model at multiple locations will preserving the dataset privacy of each facility
Working directly with Nvidia key providers we developed an Enterprise platform that is designed for Enhancing Privacy and Accelerating Discovery.
Federated learning presents a groundbreaking approach to conducting clinical trials across the 19 cancer treatment facilities and any numbers of hospitals or facilities without any patient related data ever leaving such facilities.
In traditional Machine Learning and Deep Learning approaches the datasets are uploaded to the model’s location for the latter to be trained. With Federated learning the model goes to the data and is trained where the data is housed returning back to the centralized server with gradients and weights only.
Therefore, this method allows the model to be trained on a much larger number of datasets which significantly enhances the performance of the model (20% increase in AUC with adding only a second dataset to the platform) while preserving patients privacy and anonymity.
Federated learning’s architecture inherently protects patient privacy by ensuring that raw data never leaves the hospital’s local servers.
We further augment security measure by using advanced encryption methods, utilizing VPN and ensuring that even the shared model updates are anonymized and free from sensitive information. This decentralized approach is crucial for maintaining compliance with data protection regulations, such as HIPAA, while still enabling cutting-edge research and clinical advances.
Breaking down the workflow:
– Each hospital’s data, including imaging (e.g., CT scans, MRIs), clinical records (e.g., patient histories, lab results), and pathology data (e.g., biopsy results), is pre-processed and kept within the local servers of the respective institutions.
– A global model is initialized by the central server, typically housed in a secure environment, and this initial model is sent to each hospital.
– Each hospital trains the model on its local dataset.
– Instead of sharing the raw data, each hospital sends only the model updates (i.e., the changes in model weights) back to the central server. These updates are essentially encrypted and do not contain identifiable patient information, thus preserving privacy.
– The central server aggregates the updates from all participating hospitals. Techniques such as federated averaging are used to combine these updates into a single global model.
– The global model is then updated and sent back to the hospitals for further training, improving its ability to generalize across different populations and data distributions
Add Your Heading Text Here
In a practical scenario, federated learning could be used to develop a model for the early detection of aggressive cancer types, such as pancreatic cancer, which often goes undetected until it reaches an advanced stage. By integrating imaging data, clinical data and pathology reports, the model can be trained to identify early warning signs that are often missed in routine screenings.
For instance, one hospital may have a dataset rich in imaging data but limited in pathology results, while another might have extensive clinical records but fewer imaging samples. Federated learning allows these institutions to contribute to a single model that can detect the onset of pancreatic cancer earlier than current methods by leveraging the strengths of each dataset. The model could, for example, detect minute changes in imaging that correlate with early pathological findings, guiding clinicians to intervene earlier with potentially life-saving treatments.